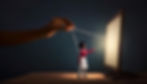
Predictive AI and Streaming
By: Nikita Sophochkin
Most of the time, we don’t open Netflix with a plan. Unless you have a show or movie you’re continuing to watch, it’s not likely that the service has something that you’ve seen on a billboard or on in a trailer: most releases on Netflix aren’t even new movies. In fact, most movies tend to only come to streaming services after 45-70 days, and some take considerably longer – by the time they become available, the hype around their release tends towards dying or dead.
Despite this, Netflix boasts a staggering 238 million subscribers, with a whopping 5.9 million joining in Q2 of 2023 alone (InfoQ). How do they pull this off? The secret ingredient isn't something flashy or headline-grabbing, like ChatGPT or DALL-E. It's in a tool you've probably used without a second thought - Netflix's recommendation system. Of course, Netflix’s AI isn’t comparable to what one expects from ChatGPT, DALL-E, or any competitor: it’s not a flashy generative bot making new movies by re-creating the likeness or voices of dead actors. It’s in something you’ve used, likely without giving it much thought – the Netflix recommendation system.
To examine its success, it’s important to have a rudimentary understanding of just what the system is. It dates back to the year 2000, when Netflix was named Cinematch. At its core, it works through a very simple system – collaborative filtering. It’s something best described via an example. Suppose a lot of people enjoyed "Knives Out", making it a sort of universal pleaser. But "The Gray Man"? Not everyone's cup of tea. If you and another viewer liked it, and that viewer also enjoyed "Bird Box", guess what's popping up on your recommendation list? That's the essence of the system that's been winning hearts since 2000 (HowStuffWorks).
It’s not, however, the only part of the system. Netflix’s success comes from something that not many other streaming services have embraced: collecting and analyzing data in virtually every single part of the process.
If you’ve ever noticed that your Netflix page looks slightly different from a friend’s, this is the result of something called A/B Testing – which is exactly what it sounds like. Two different versions of Netflix exist. You get version A, which doesn’t have a ‘Top 10 Movies’ feature. Your friend gets version B, which does. If they watch a significant number of movies from that Top 10 list, the feature gets added for everyone – or vice versa (White). The result, of course, is something that every business owner wants: experimentation with minimal risk. Instead of news articles and website rollbacks, Netflix just quickly pushes unwanted features off into oblivion, and only a small percentage of people know it ever existed. As certain business decisions show - the Metaverse comes to mind as a recent example - this form of safeguard can prevent a successful business from ‘innovating’ into catastrophic failure. Netflix even uses this system to decide which thumbnails are shown for which movies, depending on what a user has watched before.
Netflix's approach to AI has set a benchmark in the streaming industry. But it's not the only player in the game. As AI continues to evolve, other streaming services are also adopting and adapting these technologies to enhance user experiences and maintain competitiveness.
Amazon Prime Video, for instance, also employs machine learning to provide personalized recommendations. Unlike Netflix, however, Prime Video has a broader data set to draw from: Amazon's vast retail and digital ecosystem. The system can, in theory, recommend a film based on your recent book purchases or even the type of clothes you've been browsing. The interconnectivity of Amazon's world offers a more holistic understanding of a user's preferences (Forristal).
Hulu, another heavyweight, is using AI to make ads less of a nuisance for the viewer. Recognizing that advertisements can be a pain point, Hulu’s AI analyzes viewing habits to place ads at moments when they’re least likely to be disruptive. The aim is to make the advertising experience more organic and less intrusive, increasing the likelihood of an ad actually being viewed and remembered (Protocol).
But, stepping away from the giants, what about the more niche platforms? Take MUBI, for instance, a service that prides itself on curating a selection of indie and art-house films. While the selection is human-curated, AI is used to determine which films to suggest to viewers and when. This ensures that even within a more limited library, users always find something appealing to watch (Mattias).
So, with all these advancements, one might wonder: how can an aspiring student or a small developer harness the power of AI in streaming? The answer lies in open-source tools and platforms. Tools like TensorFlow and Keras have democratized access to deep learning. For those interested in building recommendation systems, platforms like MovieLens offer datasets that can be used to train algorithms. Furthermore, tutorials abound online, guiding beginners through the process of building recommendation engines akin to those used by streaming giants (Yegulalp).
Simply put, incorporating AI in streaming is undeniably critical, but it's not without pitfalls. The risk of creating "echo chambers" or "filter bubbles" is real. When AI constantly recommends content based on past behavior, users might miss out on diverse and new genres that could potentially interest them. This can lead to a homogenization of content, where viewers are repeatedly exposed to similar types of content. If everyone watches action movies, there’s no reason to recommend anything else, because the AI isn’t quite capable of understanding the idea that people are simply going to get sick of a single genre after a while. Even if one looks at it from a purely pragmatic perspective, there are also ethical considerations: data collection can be a dangerous task when it comes to gathering data that could be used to identify a customer and keeping that data secure.
That being said, for anyone willing to maneuver around such risks, the widespread open-source nature of AI makes this development a unique opening within an industry otherwise dominated by giants. Instead of trying to compete with yet another front-end website that simply links to ChatGPT, this opening leaves room for a creative entrepreneur to implement artificial intelligence directly into the foundation of a streaming service: a more unique innovation with the chance to break into a market not yet entirely saturated by the new wave of AI.
Works Cited:
“The Data-Powered Success of Netflix’s “Stranger Things.”” Www.epsilon.com, www.epsilon.com/us/insights/blog/the-data-powered-success-of-netflixs-stranger-things#:~:text=So%2C%20in%20many%20ways%2C%20Netflix.
Follows, Stephen. “How Long after Theatrical Release Do Movies Appear on Netflix?” What’s New on Netflix, 13 Oct. 2020, whatsnewonnetflix.com/news/how-long-after-theatrical-release-do-movies-appear-on-netflix/
“Managing 238 Million Memberships of Netflix: Surabhi Diwan at QCon San Francisco.” InfoQ, www.infoq.com/news/2023/10/membership-management-netflix/.
https://www.howstuffworks.com/tracy-v-wilson-author.htm, and https://www.howstuffworks.com/about-author.htm. “How Netflix Works.” HowStuffWorks, 14 May 2007, electronics.howstuffworks.com/netflix2.htm.
Chris. “Stranger Things Reveals the Real Power of Data Science.” Chris Fox Writes, 15 Aug. 2016, www.chrisfoxwrites.com/2016/08/15/stranger-things-reveals-the-real-power-of-data-science/.
White, Madeleine. “Constantly Evolving Product Offerings with A/B Testing at Netflix.” The Audiencers, 29 Nov. 2022, theaudiencers.com/inspirations/constantly-evolving-product-offerings-with-a-b-testing-at-netflix/.
Forristal, Lauren. “Amazon Brings New AI-Driven Features to Thursday Night Football.” TechCrunch, 24 Aug. 2023, techcrunch.com/2023/08/24/amazon-prime-video-ai-features-thursday-night-football/.
“Hulu Deepfaked Its New Ad. It Won’t Be the Last.” Protocol — the People, Power and Politics of Tech, 21 Aug. 2020, www.protocol.com/hulu-deepfake-commercial-ai.
Mattias Frey. Mubi and the Curation Model of Video on Demand. S.L., Springer Nature, 2021.
Yegulalp, Serdar. “What Is TensorFlow? The Machine Learning Library Explained.” InfoWorld, 6 June 2018, www.infoworld.com/article/3278008/what-is-tensorflow-the-machine-learning-library-explained.html.